Deepfake is a term used to describe the process of using artificial intelligence (AI) to create or alter video and audio content in a way that makes it appear authentic. But is actually manipulated or fabricated. It involves the synthesis and manipulation of images, videos, or audio recordings to make them appear as if they were created by someone else or to make someone say or do something they did not.
The term “deepfake” is derived from “deep learning,”
A subfield of AI that involves training neural networks on large amounts of data to recognize patterns and generate new content. Deepfake techniques typically utilize generative adversarial networks (GANs), which consist of two neural networks: a generator and a discriminator. The generator network creates fake content, while the discriminator network tries to distinguish between real and fake content. Through an iterative process, the two networks compete and improve, leading to the creation of more convincing deepfakes over time.
Deepfake technology has garnered attention due to its potential negative implications. Here are some use cases of deepfakes:
- Entertainment: Deepfakes have been used in the film industry to recreate the likeness of actors, resurrecting deceased stars or altering performances. They can also be used for creating visual effects, replacing stunt doubles, or enhancing makeup effects.
- Political manipulation: Deepfakes can be used to create fabricated videos or audio recordings of political figures, potentially causing misinformation, damaging reputations, or swaying public opinion. This poses significant challenges to the integrity of elections and democratic processes.
- Fraud and scams: Deepfakes can be employed to manipulate or impersonate individuals, such as CEOs, celebrities, or friends, for fraudulent activities. For example, scammers may use deepfake voice impersonation to deceive people into transferring money or revealing sensitive information.
- Revenge porn and harassment: Deepfake technology has facilitated the creation of non-consensual pornography, where an individual’s face is superimposed onto explicit content. This can be used for blackmail, harassment, or to damage someone’s reputation.
- Education and research: Deepfakes can be used for educational purposes, such as historical recreations or language learning. Researchers also utilize deepfake technology to understand its implications better, develop detection methods, and explore countermeasures.
Given the potential for misuse, deepfake technology raises ethical, legal, and societal concerns. The development of robust detection methods and legislation to address the negative consequences of deepfakes is an ongoing challenge to mitigate their potential harm.
Creating deepfakes that look real and authentic
Requires sophisticated techniques and careful attention to detail. Here are some common methods employed to enhance the realism of deepfakes:
- Training on large datasets: Deepfake models are trained on extensive datasets that contain diverse examples of the target person or content. The larger and more varied the dataset, the better the model can capture the nuances and characteristics of the person being manipulated.
- Facial alignment: Deepfake algorithms align the target face with the source face to ensure that they match in terms of head pose, facial landmarks, and expressions. This alignment helps to maintain coherence and realism in the final output.
- Facial reenactment: Deepfake models analyze the source and target faces to capture their facial movements, expressions, and speech patterns. By mapping the movements of the source face onto the target face, the deepfake can convincingly reproduce the target person’s actions.
- Attention to details: Realistic deepfakes pay attention to finer details like lighting conditions, shadows, reflections, and background consistency. Mimicking these details accurately helps in creating a more convincing and authentic result.
Detecting deepfakes can be challenging
but researchers are continuously developing techniques to identify manipulated content. Here are some common methods used for deepfake detection:
- Forensic analysis: Researchers analyze artifacts and inconsistencies present in deepfakes, such as unusual facial movements, unnatural blurring or distortion, mismatched lighting or shadows, or discrepancies in reflections.
- Metadata analysis: Examining the metadata associated with the video or image, such as creation date, device information, or editing history, can provide clues about its authenticity.
- Deepfake-specific detection algorithms: Researchers are developing AI-based algorithms specifically designed to detect deepfakes. These algorithms analyze patterns in the visual or audio data to identify anomalies and inconsistencies that indicate manipulation.
- Multi-modal analysis: Deepfakes often involve the manipulation of both video and audio. Analyzing the synchrony and coherence between the audio and visual components can help detect discrepancies and identify potential deepfakes.
It’s important to note that deepfake detection methods are constantly evolving, and the arms race between creators and detectors continues. As deepfake technology advances, so do the detection techniques, aiming to stay ahead of the manipulation methods.
One example of an AI-based algorithm used to detect deepfakes
Is called “FaceForensics++.” It is a popular deepfake detection framework that utilizes machine learning techniques to analyze visual patterns and identify manipulated videos. Here’s a simplified explanation of how FaceForensics++ works:
- Dataset preparation: The algorithm is trained on a large dataset that contains both real and manipulated videos. The dataset consists of various deepfake techniques, including different face swapping and reenactment methods.
- Feature extraction: FaceForensics++ extracts a set of visual features from each video frame, such as color histograms, optical flow, or noise patterns. These features capture unique characteristics and patterns that differ between real and manipulated videos.
- Classifier training: Using the extracted features, FaceForensics++ trains a machine learning classifier, such as a deep neural network or a support vector machine (SVM). The classifier learns to distinguish between real and manipulated videos based on the patterns observed in the training dataset.
- Testing and detection: When presented with a new video, the trained classifier analyzes the extracted features from its frames. It then assigns a probability score indicating the likelihood of the video being a deepfake. Thresholds can be set to classify videos as either real or manipulated based on these scores.
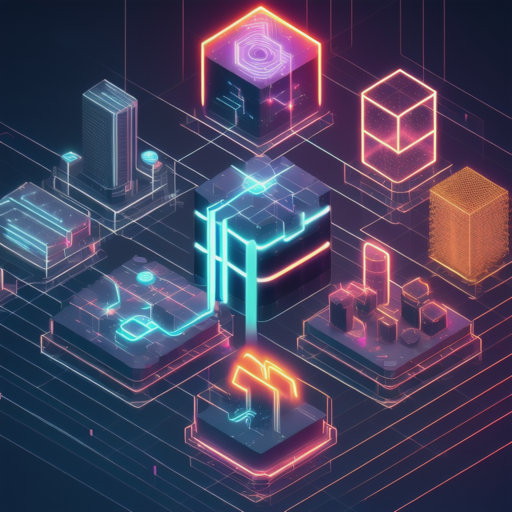
It’s important to note that FaceForensics++ is just one example, and there are various other deepfake detection approaches, each with its own unique features and methodologies. Some algorithms may focus on specific artifacts or inconsistencies introduced by certain deepfake techniques, while others use advanced deep learning architectures or employ audio-visual synchronization analysis.
Deepfake detection algorithms are continually evolving to keep up with new manipulation techniques. And researchers are actively working on improving their accuracy and robustness. However, it’s important to understand that as deepfake technology advances, so does the sophistication of the methods used to create undetectable deepfakes, resulting in an ongoing cat-and-mouse game between creators and detectors.
The prospect of deepfake detection in the future
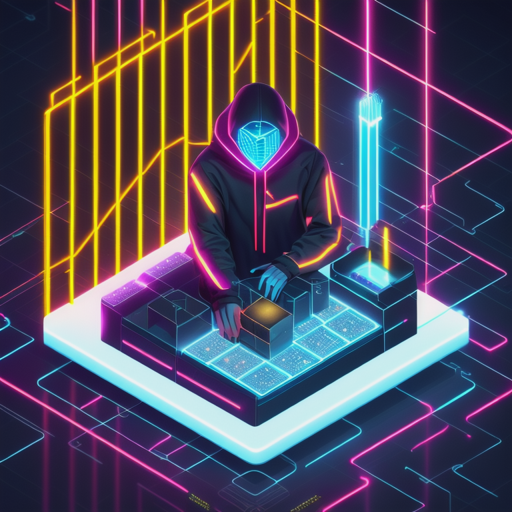
Is promising, although it is likely to be an ongoing challenge as deepfake technology continues to advance. Researchers and experts are actively working on improving detection methods, and while it may be challenging to completely eliminate deepfakes, it is possible to develop increasingly reliable techniques to identify manipulated content. Here are a few aspects that contribute to the future of deepfake detection:
- Advancements in AI and Machine Learning: As AI and machine learning techniques progress, detection algorithms can become more sophisticated and effective. Researchers are exploring advanced deep learning architectures, novel feature extraction methods, and ensemble models to enhance detection capabilities.
- Larger and More Diverse Datasets: The availability of comprehensive and diverse datasets is crucial for training deepfake detection models. As more deepfake content is created and shared, researchers can leverage these datasets to improve detection algorithms and make them more robust against new manipulation techniques.
- Multi-modal Analysis: Future detection methods are likely to focus on multi-modal analysis, combining visual and audio cues to identify inconsistencies and anomalies. Analyzing the synchronization between the visual and audio components of a video can provide additional clues to detect deepfakes.
- Deepfake Attribution and Digital Forensics: Researchers are also working on developing techniques for deepfake attribution, which aims to identify the specific methods and tools used to create a deepfake. This approach can assist in tracing the origin of manipulated content and holding responsible parties accountable.
- Collaboration and Standardization: The fight against deepfakes requires collaboration among researchers, industry experts, and policymakers. Sharing knowledge, resources, and best practices can lead to the development of standardized evaluation metrics and benchmarks for deepfake detection algorithms.
While it is challenging to predict the exact timeline or certainty of deepfake detection, it is reasonable to expect that detection methods will continue to improve. However, it is also likely that creators of deepfakes will continue to refine their techniques to make them harder to detect. Therefore, a balance between detection advancements and mitigation strategies, such as media literacy and authentication mechanisms, will be crucial in combating the negative impacts of deepfakes in the future.
Leave a Reply